It is convenient to think that by killing the head of the snake with fire and fury, the gophers will leave the garden alone. Recent history in the Middle East has shown that scenario to not only be false, but instead, the gophers reemerge more emboldened and there are holes everywhere in the garden.
Do you ever open your phone and scroll through a news feed after pressing shuffle on Spotify, before moving on to flicking across the Netflix lineup for something to pass the time? Video stores, music stores, libraries and the newspaper have been reduced to a feed. What appears to be a vast sea of information and entertainment available at the click of a button is instead spoon-fed to you by a statistical formula called a recommender system. Recommender systems are in theory designed to recommend content you may like based on content you have consumed. In practice, recommender systems hamper creative exploration and reinforce ideological entrenchment by myopically evaluating your digital activity and categorizing your interests.
After briefly describing how recommender systems function, I explore their pitfalls. My central argument is that we must vigilantly govern digital platforms’ recommender systems because they serve as the go-to sources of information and entertainment—setting the outer limits of creative exploration and truth seeking. Because access to information and art is critical to democracy in a modern society, the gateway platforms must be transparent in their methodology and allow users more choice. Recommender systems based on algorithmic transparency and user input can realize the unfilled potential of the internet by bringing the world’s content to our fingertips. I write not as a nostalgic luddite longing for the return of video stores and Walter Cronkite, but as a concerned citizen who wants the digital age to encourage creative exploration and ideological exposure.
Inside the Recommender Black Box
Recommender systems record what content users consume and apply statistical formulas to determine what they are probably willing to engage with. It is important to recognize that recommendations are not always explicit under category listings titled “recommended for you.” They are the very ordering of the movie titles and news articles you see on your phone and computer every day.
There are two main recommender formulas which platforms commonly employ to recommend content: content-based filtering and collaborative filtering. Content-based filtering takes examples of what you like and dislike (comedy films, female lead vocals, news about COVID-19 cures) and matches you with similarly categorized content. Collaborative filtering matches your preferences with people of similar consumption patterns and recommends you additional content that those people have consumed.
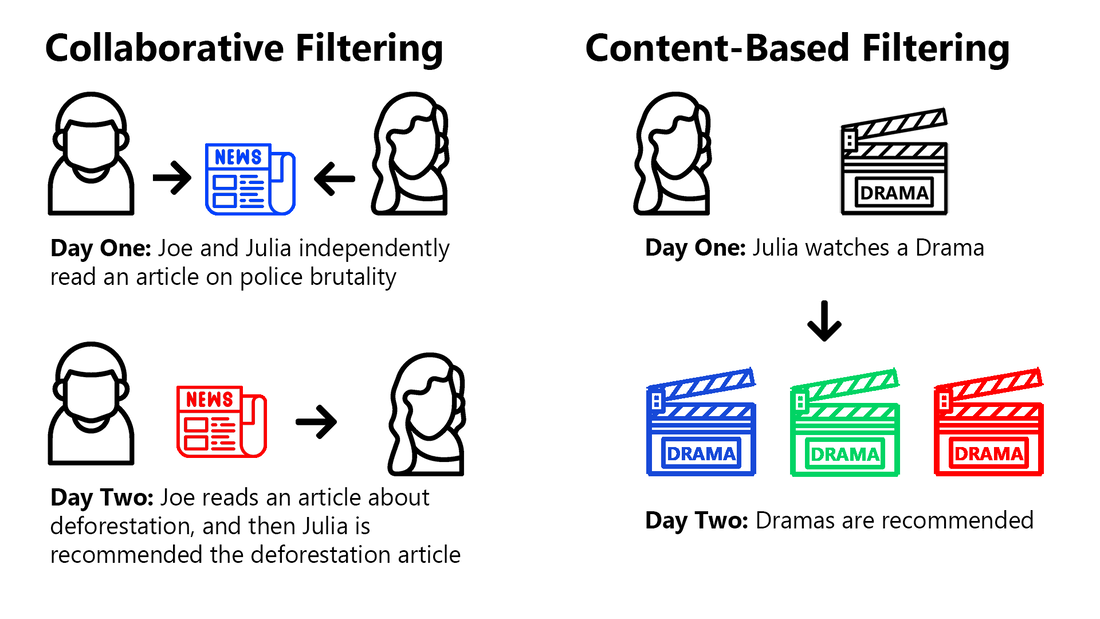
Both collaborative filtering and content-based recommender systems use what you have consumed as a proxy for what you like. They interpret your interactions on the platform, and sometimes combine that data with your interactions on other parts of the internet, forming a profile of your preferences. For example, one method is to observe which videos you click on and finish as a proxy for which videos you prefer. A better method would involve rating videos upon completion. An even better system would require a rating and reason why you did or did not like it, but participation in those surveys is spotty. Thus, actions such as playing a song twice and slowly scrolling through an article serve as workable proxies for what you like.
Problem One: Privacy & Control
Although this method of preference gathering is imperfect (what if you were cooking and could not skip a song?), the more you consume, the better the platform can determine what you consistently will engage with. By adding data from outside the platform itself, the mosaic of your preferences gets more complex, forming a profile of your defined tastes and predictable ones. Generally, the more data on your preferences the algorithm has as an input, the more tailored of a recommendation it can make. That is a slippery privacy slope, as an enormous data profile can be amassed over time by observing your habits in an effort to order your newsfeed or Spotify playlist.
Taken to the extreme, the best recommender would be inside your head, monitoring your thought patterns. We are already half-stepping towards this today. Smart speakers listen to conversations, camera embedded smart TVs can record reactions to content, web scrapers devour social media feeds and online affiliations, and Google logs internet searches. If gone unchecked, the level of psychological exploitation will inevitably grow to capture passing unexpressed thoughts, and pinpoint what type of stimuli makes you happy, sad, and makes you want to continue consuming. The more news, music, movies, and shopping platforms dominate our attention, computers and phones turn from tools into content shepherds that subtly steer us involuntarily.
What that means in practice is that we are ceding the right to filter information and media content to the companies that run the major content platforms. The complexity of these recommendation systems occults that there are people behind the wheel of the algorithms they employ, even if their systems are semi-autonomous. If psychological profiles developed for the purpose of recommender systems were to be shared with governments, employers, and schools this same preference data could be used to discriminate and manipulate. Personalized profiles could become the basis of institutional access and job opportunities, not to mention the potential for psychological manipulation of the population at large.
By sharing all of your platform usage and content choices, you are accepting that a picture will be painted of you that may or may not represent you, and you will see content through a lens you will not know was prescribed for you.
Problem Two: Loss of Uniqueness
Collaborative filtering does not consider people to be unique. By design, it attempts to match users with other users who share similar consumption patterns. However, there are preferences, such as those based on unique life experiences that are not shared with anyone on the planet. Even if you share 95% of your consumption in common with another profile, would you not prefer the freedom to scan the random content universe when you go searching for creative inspiration on Spotify, or the truth in your news feed, as opposed to being matched with your 95% consumption twin? Collaborative filtering makes the 95% similar person’s consumption habits the entire universe of news, music and shows available on the screen.
Consider that your friends are likely not anywhere near 95% similar to you in consumption patterns, and yet they can serve as an excellent source of recommendations. Why? Take news articles for example. You may disagree with a friend about a certain political candidate, but you may also be interested in the normative arguments they make by analogy to their life experience growing up in a small town in Mississippi, and you may be intrigued by the factual evidence they cite in favor of tax breaks to big businesses as a means to generate economic growth. That builds trust in their recommendation. Based on their analysis, you might be willing to read an article they recommend which discusses an instance of when big business tax breaks led to a boom in economic growth and the rise of certain boomtowns. You can evaluate the value of your friend’s recommendation considering what you know about them, as well as your knowledge of history and economics.
Collaborative filtering builds no such credibility of authority nor offers a synopsis of its reasoning. It removes the rationale from the recommendation. The collaborative filtering universe of options does not expand unless your digital twin searches for something outside of their previously viewed content. In other words, relying on collaborative filtering for recommendations ensures there are no hidden gems in your shuffle playlist or diversity of opinion in your newsfeed unless your soul sister from the ether searches for something outside of the recommender system.
Problem Three: Preference Entrenchment
Content-based recommender systems can broaden the collaborative filtering universe of recommendations by going beyond your consumption twins, but they myopically focus on the categorical characteristics of content you previously consumed. By doing so, content-based recommenders entrench you in your historical preferences, leaving no room for acquiring new tastes or ideas. Consider that if you have only ever listened to electronic dance music on Spotify, you will be hard pressed to get a daily recommendation of jazz. More likely, your playlist will be a mile-long list of electronic dance music. To get recommendation of a new type of music, you have to search for it. Unlike record shopping of old, you would never see the album art that caught your eye on your way to the electronic music section, or hear a song playing in the background of an eclectic record store.
This preference entrenchment problem has deleterious consequences in the dissemination of information in the news. For example, a frequent New York Times reader who only clicks on anti-Donald Trump articles is likely only to be recommended more articles critical of Donald Trump. There are no easily accessible back page articles in a newsfeed. The recommender system does not allow for an evolution of political views because it is only looking at users’ historical preferences. That is a problem because one may not yet have formed an opinion on something one has not been exposed to. By tagging what you consume categorically, you are being involuntarily steered and molded into categories that may not represent your interests now, or in the future.
Problem Four: Hyper-Categorization of Preferences
Preferences are not always so clear cut. Data scientists love to define more granular categories of content to pinpoint preferences. The architect of these feature-definers is often a data scientist who occasionally may be aided by an expert in the field. The data scientist’s objective is to train a machine learning algorithm that automatically categorizes all the content on the platform. For example, Spotify might employ a musician to break down songs by genre, musical instruments, tempos, vocal ranges, lyrical content, and many other variables. The data scientists would then apply those labels to the entire music library by utilizing an algorithm designed to identify those characteristics, occasionally manually spot checking for accuracy.
But, are those categories the reasons why you like a given love song? Or might the lyrics have reminded you of your ex-girlfriend or grandmother? The mechanical nature of breaking content down into feature categories often weighs superficial attributes over the ones we really care about. The flaw in the mechanical approach is subtle: by focusing on the qualities of the grains of sand (song features), recommenders fail to recognize they combine to form a beach.
In context, a great movie is not great merely because it is slightly different than another with a similar theme and cast. It is great because of the nuanced combination of emotional acting, a complex story, evolving characters, and a climax no one could have anticipated. Similarly, local artists are not merely interesting because they are not famous. Their music might be interesting because their life influences and musical freedom make their music rawer than what is frequently listened to on Spotify. Those are difficult characteristics to describe for a human, let alone a recommender algorithm. Recommenders struggle to categorize descriptions like “beautiful,” “insightful,” and “inspiring” because they are descriptions of complex emotions. Thus, their bias towards clear-cut categories and quantifiable metrics makes them poor judges of art.
It is no surprise then why recommenders are horrible at disseminating information. News articles can be broken down based on easy to identify categories such as publication source, quantity of links to other articles (citation count) or mentions of politicians’ names. Those categories might loosely relate to quality and subject, but they would hardly indicate a reason to base a recommendation. Moreover, these attributes do not signal the relevant attributes in news such as truthfulness, good writing, humor or demagoguery.
Because news is constantly changing, it is even harder to categorize than a static environment like in music or movies, which can be manually tagged ahead of time. Facebook, Google and Twitter are in an ongoing battle to tag factual accuracy in news articles, particularly because it involves large teams of real people Googling what few credible information sources remain (instead of an algorithm trained to detect the presence of a trumpet in a song). The data scientists employed by these platforms seek to automate categorization whenever possible, but when truth lies in the balance, the stakes are too high. And yet, with screening an ever-growing amount of information, the platforms are struggling to keep up, especially in complex subjects like COVID-19 cures, which require expertise to comprehend. At present, we are allowing recommender systems, aided by data scientists and contract-employee category taggers, to shape public perception. They do not appear to be helping expand access to information.
Problem Five: The User Interface is The Content Universe
Shifting from retail stores and newspapers to screen scrolling may have kept us from leaving the sofa, but it did not make finding new content altogether a better experience. Strolling through music and video stores of old allowed for quickly browsing spines and close inspections of covers, and the sheer physical nature of the act made it feel more intentional. The advantages of the physical browsing experience are why DJs still love record stores and intellectuals will not let bookstores die. The face-out titles were the curated and popular ones. The more obscure titles were deeper in the stack. It may seem counterintuitive, but the inconvenience of digging made finding the hidden gems more rewarding.
Although the subscription all-you-can-consume business model conveniently allows for casual previews and skim reading, one can only finger swipe through movie titles and cover pictures for so long before clicking. Thus, the order of songs, movie titles and articles is highly influential. Clickbait media works because of the equal weighting of articles in a scroll feed. Tabloid news magazines used to stand out with their highlighter colors next to the candy bars in the checkout line at the supermarket. Today, they often appear at least as often as the New York Times or the Economist in your feed. Recommender systems will reinforce clickbait tabloids over long form journalism without batting an eye simply because they are more frequently clicked on.
Digital platforms fail to recognize that there is a degree of stewardship in curating news content. The content universe is—and always will be—curated. The status quo means trusting the data scientist architects and their recommender black boxes that influence what you see when you read the news with your morning cup of coffee, sit down at the end of a long day to enjoy a movie, or turn press shuffle to zone out while working. Moreover, it also means accepting that the elaborate apparatus of data collection of your preferences should continue to the extreme of understanding your psychological programming and turn the digital universe into a happy pill time drain—or worse.
It is important to note that the biases of recommender systems are not always intended consequences. They are in part limitations of black box algorithms too often left unsupervised or under scrutinized. Engineering oversights happen. Applying recommender systems to the structuring of newsfeeds shifted the way a large portion of the population sees an issue like the viability of vaccines, but that was not likely the platform architects’ intentions. Nonetheless, it is the result of neglect of platform engineers, managers, and executives. The more authority over the flood gates of information are reduced to mathematical formulas contained in black box closed sourced programs, the more likely it is for this neglect to occur.
It is vital that we push back to gain control of the digital universe. It is a misconception to think that we have a world of information and content in our pockets if every scroll is based on a recommender system backstopped by a small team of people screening fringe content. The internet dominated by platforms is creating shepherds, not moral stewards. We must subjugate platforms, and their algorithms, to democratic governance and require that they be transparent in their processes to ensure that the potential for creative exploration and dissemination of truth is enhanced by their emergence as a pillar of modern life.
Democratic Platform Stewardship: An Alternative to Recommender Systems
I do not intend to suggest that the internet should merely look like the back shelf of the library. If instead of being steered by recommender systems, users are given the reigns to select their preferences, the system becomes a useful tool instead of a dictator of preferences. The categories recommenders use could easily be made available to users. Instead of endlessly harvesting data on users’ habits to feed recommender systems, users could select and choose their own preferences. In the interest of privacy, platforms could be required to not record those preference settings. The common big tech response is that the data they collect helps makes services cheaper. Privacy and control are worth a few extra dollars a month.
Another solution is altogether more democratic. Users could score content for quality, truthfulness, and other relevant categories for the medium. For a more intimate rating system, users could opt into friends and interest groups to get community recommendations that may deviate from popular opinion or involve groups of credentialed critics. Smaller community discussion could provide useful background information and prevent majority groupthink and interested parties from dominating the narrative. These groups are prone to becoming self-isolating echo chambers, so it is important that they remain public. Encouraging discussions would remove us from the infinite scroll of provocative images and titles pushed by advertisers and reinforced by recommender systems.
Newsfeeds require particular attention because they are key sources of information for many. One of the big takeaways from the Cambridge Analytica and Russian election interference scandals was that the propaganda that gained the most interest was found to be that which promoted ideological extremes and reinforced scapegoats. News feeds today are too much driven by an advertising model based on click-through rates and comment engagement, to the detriment of critical thinking and the dissemination of truth. Recommender systems left to their textbook formulas may be good for engagement but are bad for the spread of truth. That is not acceptable.
One potential solution in newsfeeds is to have community-based experts score each news posts for truthfulness and have users score for ideology, with a tiered system of users to include community elected expert moderators whose scores are given extra weight. The presence of moderators can provide a check on exploitation by any party who might seek to influence the platform by voting with fake accounts. All news feeds should seek to be balanced in ideology, but always attempt to be truthful. Balancing ideology aims to properly give readers both sides of a given issue. Bias may be impossible to eliminate, but stewardship in the curation of the modern newspaper is essential. Balanced journalism, however difficult to achieve, must at least be strived for in the digital age.